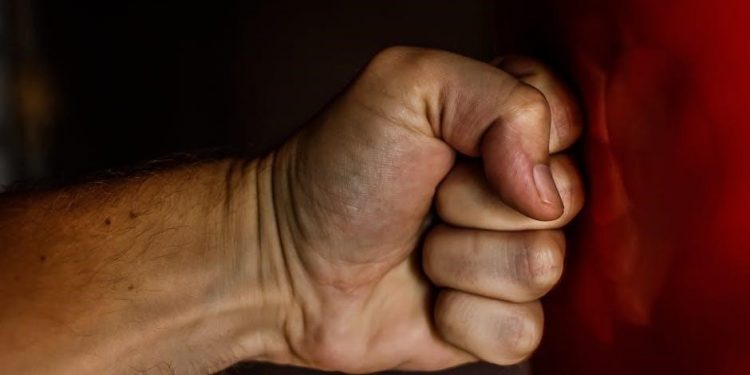
Overview of FIST
The Function in Sitting Test (FIST) is a 14-item, performance-based clinical assessment of sitting balance, designed for administration by healthcare providers at the bedside, typically within 5-10 minutes.
1.1 Definition and Purpose
The Function in Sitting Test (FIST) is a 14-item, performance-based clinical assessment designed to evaluate sitting balance in patients. Developed by Sharon L. Gorman, it is administered by healthcare providers, primarily physical therapists, at the bedside. The test aims to measure a patient’s ability to maintain balance while performing specific tasks, such as reaching or responding to external nudges. Its purpose is to identify impairments in sitting balance and monitor progress during rehabilitation, particularly for patients with limited mobility or neurological conditions. The FIST is concise, typically lasting 5-10 minutes, making it practical for clinical settings.
1.2 Key Features of the FIST
The Function in Sitting Test (FIST) is a 14-item, performance-based assessment evaluating sitting balance through specific tasks like reaching and responding to external nudges. It is administered by healthcare providers, such as physical therapists, and typically lasts 5-10 minutes. The test is designed for bedside use, making it practical for clinical settings. Its standardized approach ensures consistency in measuring balance impairments and tracking patient progress during rehabilitation.
Clinical Application of FIST
The Function in Sitting Test (FIST) is a clinical tool used to assess sitting balance in patients, aiding in rehabilitation and therapy by identifying specific balance impairments.
2.1 Purpose of the Function in Sitting Test
The Function in Sitting Test (FIST) is designed to evaluate sitting balance in patients, providing insights into their ability to maintain posture and perform functional tasks while seated. It helps healthcare providers identify specific balance impairments and monitor progress during rehabilitation. The test is particularly useful for patients with limited mobility or those in early stages of recovery, guiding targeted therapeutic interventions.
2.2 Administration and Duration
The Function in Sitting Test (FIST) is administered by a healthcare provider, typically at the bedside, and takes approximately 5-10 minutes to complete. The test involves 14 items assessing sitting balance and can be adapted based on patient needs, such as repositioning or additional support. Its concise design ensures efficiency while providing valuable insights into a patient’s functional abilities, making it practical for clinical settings.
FIST Training and Instruction
The Function in Sitting Test (FIST) Training and Instruction Manual, developed by Sharon L. Gorman and funded by the California Physical Therapy Fund, provides detailed training for healthcare providers.
3.1 Development and Funding
The Function in Sitting Test (FIST) was developed by Sharon L. Gorman, PT, DPTSc, GCS, with funding from the California Physical Therapy Fund (grant 05-01). The grant supported the development and pilot testing of FIST. The training manual, version 1.5, was finalized on May 20, 2011, providing standardized administration guidelines for healthcare providers.
3.2 Training Manual and Content
The FIST Training Manual provides detailed guidelines for administering the 14-item assessment, ensuring standardized evaluation of sitting balance. Developed by Sharon L. Gorman, the manual includes instructions for test item administration, scoring, and interpretation. It emphasizes content validity, reliability, and practicality for healthcare providers, serving as a comprehensive resource for consistent and accurate FIST implementation across clinical settings.
Methodology and Validation
The FIST methodology involved a study approved by Institutional Review Boards, with 17 therapists rating videos of 7 participants, ensuring test-retest reliability and validity.
4.1 Study Design and Approval
The FIST study was approved by the Institutional Review Boards of San Francisco State University and Alta Bates Summit Medical Center. It involved 17 therapists rating videos of 7 participants, with a two-week break between test and retest administrations to ensure reliability. The methodology focused on assessing internal consistency, content validity, and construct validity, laying a robust foundation for clinical application and further research.
4.2 Internal Consistency and Validity
The FIST demonstrated strong internal consistency, with a Cronbach’s alpha of 0.97, indicating high reliability among its 14 test items. Content validity was established through expert review, ensuring items accurately assessed sitting balance. Construct validity was confirmed by correlating FIST scores with other balance measures, while inter-rater reliability was high (0.95), confirming its effectiveness as a consistent and valid clinical assessment tool.
FIST in Rehabilitation and Therapy
FIST is a valuable tool in rehabilitation, tracking progress and boosting patient confidence. It aids in improving sitting balance and functional abilities in diverse patient populations.
5.1 Case Studies and Patient Outcomes
Case studies demonstrate FIST’s effectiveness in rehabilitation. For example, Mary, a 70-year-old with Parkinson’s, showed improved reaching abilities post-FIST assessment. Patients with balance challenges achieved better sitting stability and confidence. FIST’s clear documentation of progress motivates patients, enhancing their engagement in therapy and overall recovery outcomes.
5.2 Improvements in Patient Confidence
The FIST provides measurable progress, enhancing patient confidence. For instance, a patient’s FIST results showed significant improvement, boosting their belief in therapy effectiveness. This visible progress fosters motivation, leading to increased participation and better overall outcomes in rehabilitation.
The Role of FIST in Parkinson’s Disease Management
FIST is a valuable tool in managing Parkinson’s disease, assessing specific motor challenges like reaching. It provides insights to tailor therapy, improving functional abilities and patient outcomes effectively.
6.1 Challenges in Motor Function
Parkinson’s disease presents significant motor function challenges, including impaired balance, coordination, and reaching abilities. FIST effectively identifies these deficits, particularly in tasks requiring forward and lateral movements. By assessing sitting balance and postural stability, FIST provides actionable insights to address specific motor impairments, enhancing therapeutic interventions and improving patient mobility and confidence in daily activities.
6.2 Therapeutic Insights from FIST Results
FIST results provide valuable therapeutic insights, particularly for Parkinson’s patients. By identifying specific motor challenges, such as difficulties in reaching or postural stability, FIST guides personalized interventions. Tracking progress over time, FIST enhances understanding of treatment effectiveness, enabling clinicians to refine therapies. These insights not only improve mobility but also boost patient confidence, fostering greater engagement in rehabilitation efforts and overall disease management.
FIST as a Machine Learning Methodology
FIST applies machine learning for design flow parameter tuning, leveraging feature-importance sampling and tree-based methods to optimize complex systems efficiently and dynamically.
7.1 Feature-Importance Sampling and Tree-Based Tuning
FIST employs feature-importance sampling to identify critical parameters and uses tree-based methods to optimize design flow tuning. This approach efficiently captures parameter impacts, enabling dynamic adaptation and improving learning accuracy in complex systems. The integration of XGBoost models and dynamic tree techniques enhances the methodology’s robustness and scalability for advanced parameter optimization tasks.
7.2 Applications in Design Flow Parameter Tuning
FIST is applied in design flow parameter tuning to optimize chip design quality. Its automatic tuning process reduces manual effort and speeds up complex design flows. By leveraging feature-importance sampling, FIST efficiently handles large search spaces and enhances the accuracy of parameter effects, making it a valuable tool in modern design flow optimization.
Advantages of the FIST Method
The FIST method excels in efficiency, dynamically adapting to complex systems and optimizing parameter learning. Its automated tuning process enhances accuracy and reduces manual effort significantly.
8.1 Efficiency in Parameter Learning
The FIST method demonstrates exceptional efficiency in parameter learning, leveraging Bayesian methods and XGBoost models to dynamically adapt to complex systems. By reducing manual tuning efforts, it streamlines the process, ensuring accurate and timely results. Its ability to handle intricate design flows and chip design parameters makes it a valuable tool for optimizing performance in various applications.
8.2 Dynamic Adaptation in Complex Systems
FIST excels in dynamically adapting to complex systems, enabling efficient parameter tuning across diverse applications. Its machine learning methodology, incorporating XGBoost models, allows seamless transitions between traditional CGRA and neural network configurations. This adaptability ensures optimal performance in design flows, making FIST a versatile tool for handling intricate system requirements and enhancing overall design quality.
Challenges and Limitations
FIST faces challenges in complex implementations, requiring specialized expertise. Its dynamic nature demands a unique approach to failure analysis, differing from traditional methodologies.
9.1 Complexity in Implementation
The FIST methodology presents challenges due to its dual application in clinical and machine learning contexts. Clinicians require expertise in administering the 14-item test accurately, while data scientists need proficiency in parameter tuning and tree-based algorithms. The complexity lies in balancing precise test administration with advanced computational techniques, making it demanding for practitioners to master both domains effectively.
9.2 Comparison with Traditional Methods
FIST offers advantages over traditional methods, including efficiency in parameter learning and dynamic adaptation. However, its implementation requires specialized expertise, contrasting with simpler, more straightforward conventional approaches. While FIST excels in complex systems, traditional methods remain effective for routine applications, emphasizing the trade-off between sophistication and accessibility in clinical and computational contexts.
FIST has proven impactful in rehabilitation and therapy, enhancing patient confidence and outcomes. Future research should explore its integration with emerging technologies and expanded clinical applications.
10.1 Summary of FIST’s Impact
The FIST has demonstrated significant effectiveness in assessing sitting balance, proving invaluable in rehabilitation settings. It has shown notable improvements in patients with Parkinson’s disease, enhancing their functional abilities and confidence. The test’s concise administration and clear outcomes make it a reliable tool for healthcare providers, contributing meaningfully to patient recovery and therapeutic success.
10.2 Potential for Future Research and Development
Future research could explore expanding FIST’s applications beyond sitting balance, such as integrating it with emerging technologies like wearable devices or AI for enhanced accuracy. Additionally, investigating its effectiveness in diverse patient populations and settings could broaden its clinical utility. Developing advanced training modules and digital tools could further enhance its accessibility and reliability for healthcare providers globally.